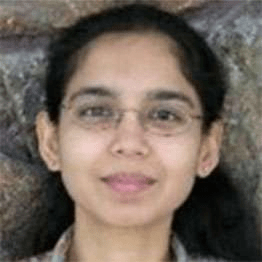
Bhuvana Ramabhadran
Google research, USA
A Broad Perspective on Large Scale Self/Semi-Supervised Learning for Speech Recognition
Supervised learning has been used extensively in speech and language processing over the years. However, as the demands for annotated data increase, the process becomes expensive, time-consuming and is prone to inconsistencies and bias in the annotations. To take advantage of the vast quantities of unlabeled data, semi-supervised and unsupervised learning has been used extensively in the literature. Self-supervised learning, first introduced in the field of computer vision, is used to refer to frameworks that learn labels or targets from the unlabeled input signal. In other words, self-supervised learning makes use of proxy supervised learning tasks, such as contrastive learning to identify specific parts of the signal that carry information, thereby helping the neural network model to learn robust representations. Recently, self-supervised (pre-training) approaches for speech and audio processing that utilize both unspoken text and untranscribed audio are beginning to gain popularity. These approaches cover a broad spectrum of training strategies such as, unsupervised data generation, masking and reconstruction to learn invariant and task-agnostic representations, and at times even guiding this pre-training process with limited supervision. With the advent of models pre-trained on diverse data at scale that can be adapted to a wide range of downstream applications, a new training paradigm is beginning to emerge with an increased focus on utilizing these pre-trained models effectively. This talk provides an overview of the successful self-supervised approaches in speech recognition with a focus on methods to learn consistent representations from data through augmentation and regularization, utilize predictions from synthesized speech and algorithms to complement the power of contrastive learning to jointly learn from untranscribed speech and unspoken text.
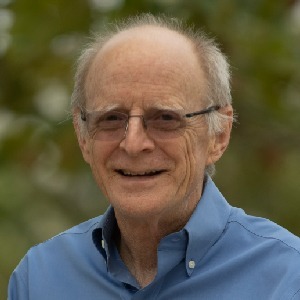
James W Pennebaker
UT Austin
Natural language in natural environments
The words people use in everyday life reflect their social and psychological worlds. Across languages and cultures, the analyses of texts from conversations, emails, speeches, and other genres can help us understand individual, group, and even societal shifts. A large number of studies will be summarized that rely on word counting methods that are linked to hard social and behavioral outcomes. The presentation concludes with a call for a greater understanding of fundamental social and psychological processes that are driving and reflecting word usage.
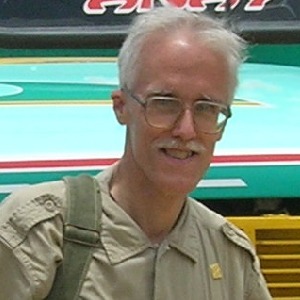
John M. Lipski
The Pennsylvania State University
The Palenquero language: overview, basic structures, and perspectives for ASR
Palenquero (known locally as Lengua ri Palenge ‘[the] language of Palenque’) is a Spanish-lexified Afro-Atlantic creole language, spoken together with Spanish in the Afro-Colombian village of San Basilio de Palenque. Palenquero emerged around the turn of the 18th century when enslaved Africans fled from the port of Cartagena and established fortified villages in rural regions to the south. The lexicons of the two languages are more than 90% cognate, ranging from complete identity (based on the local vernacular variety of Spanish) to predictable phonological modifications resulting from the historical development of Palenquero in contact with Kikongo and other Central African languages. However due to significant grammatical differences, including the lack of subject-verb and adjective-noun agreement and restructured verbal and negation systems, Palenquero and Spanish are not mutually intelligible. To further complicate matters, Palenquero speakers sometimes introduce Spanish-like elements, ranging from conjugated verbs and preverbal clitics to more complex morphosyntactic constructions, but do not consider these items to be intrusions, interference, or code-switching (Lipski 2016a, 2016b). Previous research (Lipski 2020) has demonstrated that in online identification of the language(s) of an utterance, Palenquero-Spanish bilinguals are influenced by key grammatical items as well as by regular Palenquero-Spanish phonotactic correspondences, but this presupposes simultaneous activation of both languages. The complexities of the Palenquero-Spanish interface thus provide a unique challenge for automatic speech recognition. This presentation describes the sociolinguistic environment in which Palenquero is spoken, gives an overview of basic phonetic and grammatical patterns, and offers preliminary points to be considered when developing automatic speech recognition of Palenquero.
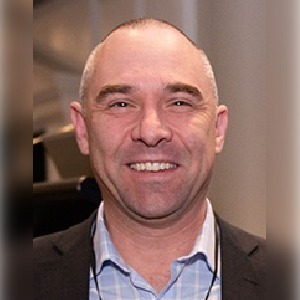
Julian Epps
University of New South Wales, Australia
Recognition of Depression from Speech: From Fundamentals to Mobile Devices
Speech production represents the most complex coordination of neuromuscular activity in the entire body, and is therefore sensitive to a vast range of influencing factors, including mental health. With a recent US Census Bureau survey finding that more than 42% of respondents experienced symptoms of anxiety or depression, there is a clear need for automatic early detection methods, however as a research problem there are a surprising number of differences from other speech recognition problems. This presentation focuses on the characterization and modeling of depressed speech, acoustic feature extraction, different possible system designs and recent promising approaches, together with how these might be used in the context of screening or clinical applications. Prospects for contributions from the speech recognition literature will also be discussed. Since speech can be conveniently collected non-intrusively at large scale and low cost via smartphone, the resultant challenges of unwanted variability in the signal and interesting opportunities for elicitation design will be covered. Finally, some exciting horizons for future research in depression recognition and related problems will be suggested.
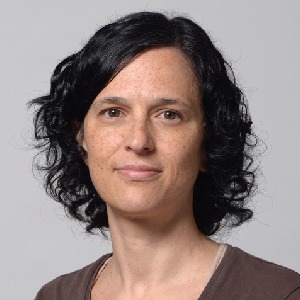
Luciana Ferrer
Universidad de Buenos Aires, Argentina
The importance of Calibration in Speaker Verification
Most modern speaker verification systems produce uncalibrated scores at their output. That is, while these scores contain valuable information to separate the two classes of interest (same-speaker and different-speaker), they cannot be interpreted in absolute terms, only relative to their distribution. A calibration stage is usually applied to the output of these systems to convert them into useful absolute measures that can be interpreted and reliably thresholded to make decisions. In this keynote, we will review the definition of calibration, present ways to measure it, discuss when and why we should care about it, and show different methods that can be used to fix calibration when necessary. While the talk will focus on the speaker verification task, much of what will be described applies to any binary classification task.
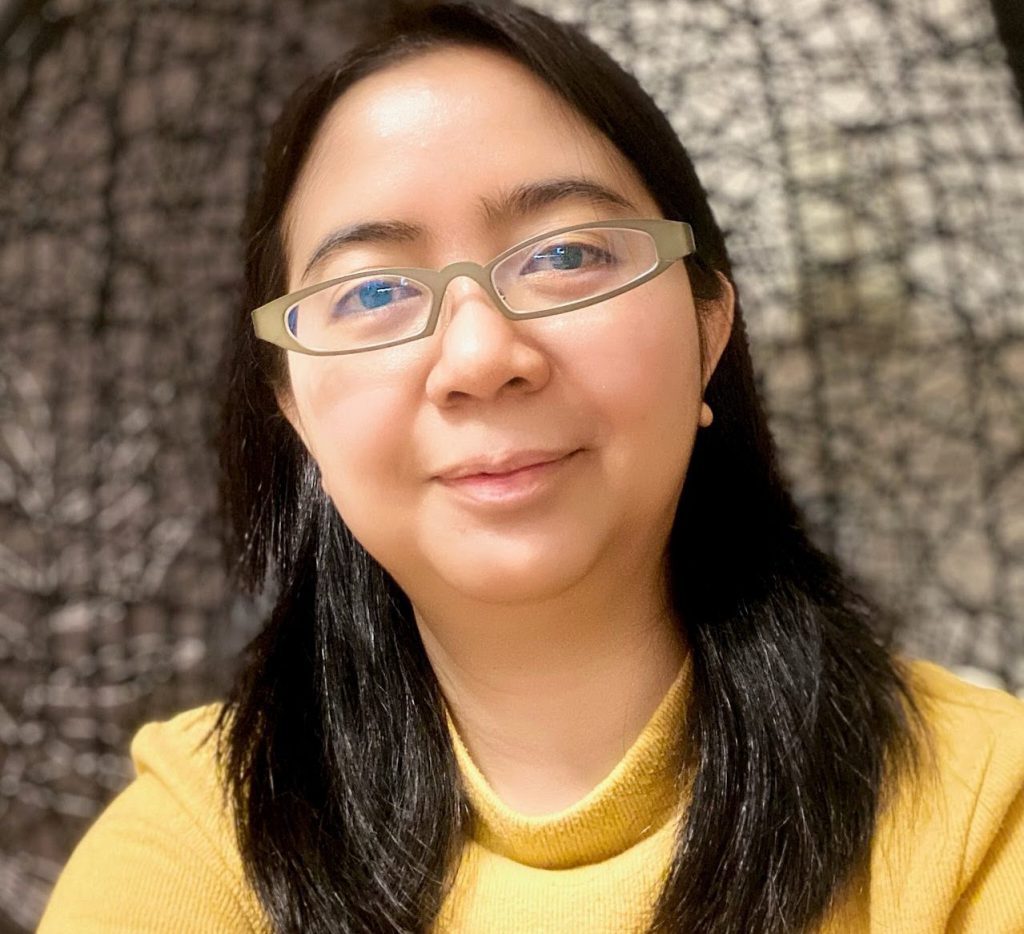
Sakriani Sakti
Japan Advanced Institute of Science and Technology (JAIST) / Nara Institute of Science and Technology (NAIST)
Machine Speech Chain: A Deep Learning Approach for Training and Inference through Feedback Loop
The development of automatic speech recognition (ASR) and text-to-speech synthesis (TTS) has enabled computers to learn how to listen or speak. However, computers still cannot hear their own voice, as the learning and inference to listen and speak are done separately and independently. Consequently, the separate training of ASR and TTS in a supervised fashion requires a large amount of paired speech-text data—furthermore, there is no ability to grasp the situation and overcome the problem during inference. On the other hand, humans learn how to talk by constantly repeating their articulations and listening to the sounds produced. By simultaneously listening and speaking, the speaker can monitor her volume, articulation, and the general comprehensibility of her speech. Therefore, a closed-loop speech chain mechanism with auditory feedback from the speaker’s mouth to her ear is crucial. In this talk, I will introduce a machine speech chain framework based on deep learning. First, I will describe the training mechanism that learns to listen or speak and to listen while speaking. The framework enables semisupervised learning in which ASR and TTS can teach each other given unpaired data. Applications of multilingual and multimodal machine speech chains to support low-resource ASR and TTS will also be presented. After that, I will also describe the inference mechanism that enables TTS to dynamically adapt (“listen and speak louder”) in noisy conditions, given the auditory feedback from ASR.